Answer any question in any channel
SupportLogic + xFind provide precise answers to complex technical questions for your end customers and employees using your existing data sources.
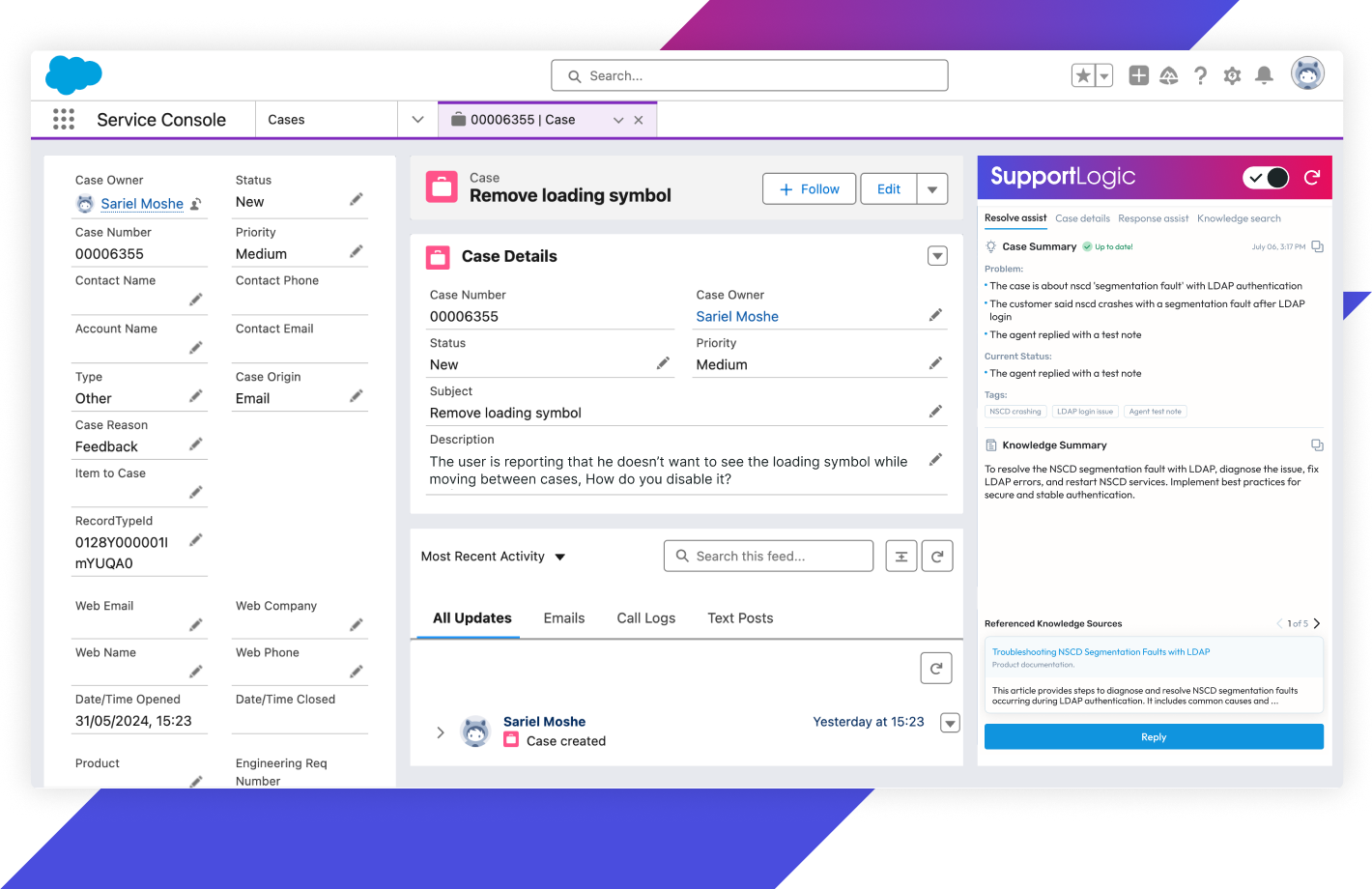
Knowledge Copilot automatically suggests answers to cases in real time.
Connect your systems of record and power your answer portal with the highest level accuracy.
Reduce case volume and empower your chatbot with fast, accurate knowledge in real-time.
Liberate knowledge across your company and get answers to any complex questions.
How Knowledge Copilot Works
SupportLogic takes a natural language question or converts an existing support case into a natural language question using advanced GenAI summarization. It then scans all connected data sources to find the relevant information and serves the most precise answers.
Why Common RAG Doesn’t Work
Common RAG cannot handle the data source complexity of support cases and customer queries. The result: search inaccuracy. Pre-trained embeddings from build-it-yourself methods are not accurate on domain-specific data.
Precision RAG is the Answer
Precision RAG consistently prepares the data from any source for the process of precise question-answering, to make use of a wide variety of retrieval methods for finding the most relevant knowledge, analyzing only the information required to produce an answer.
The proprietary guardrails in xFind’s technology automatically evaluate whether a query is relevant to the knowledge domain and if the sources can provide an accurate answer. This helps minimize errors related to topic mismatches or missing data, ensuring more reliable and accurate responses.
Precision RAG Outperforms Industry Benchmarks
In complex support, the main sources of knowledge will not be an organized knowledge base but instead past cases. This type of data cannot simply be thrown at a LLM if you’re expecting consistency. This fact can be seen in the benchmark data below, comparing us to an OpenAI embedding in retrieval of internet-available vs. domain-specific data scenarios (March 2024).
Data type | Collection | Success@5 ADA-2.0 | Success@5 SupportLogic | Failure@5 ADA-2.0 | Failure@5 SupportLogic | No. of Queries | No. of Documents |
---|---|---|---|---|---|---|---|
Public | Ember | 91% | 96% | 9% | 4% | 102 | 123 |
Slightly technical | Owllabs | 91% | 94% | 9% | 6% | 250 | 694 |
Technical | 8X8 | 75% | 86% | 25% | 14% | 250 | 3,227 |
Domain specific | Waters | 78% | 87% | 22% | 13% | 400 | 13,504 |
Take a Tour
Convert existing knowledge into precise answers
When you connect your CRM, chatbot, and issue-tracking systems under one unified knowledge copilot, you enable performance that far exceeds a single CRM’s answering capabilities.
Frequently Asked Questions
CRM knowledge management is often constrained to the information within your CRM instance. xFind’s knowledge copilot collates any number of CRMs and brands of CRM, plus bug-tracking software and any other information sources.
This brand-agnostic approach ensures that all points of information are included in the data pool when serving answers to your support team and customers.
Knowledge Copilot indexes the relevant enterprise knowledge sources and, using a proprietary engine, retrieves the items holding the information relevant to answering a given question and generates an answer while running through quality guardrails to ensure relevance and correctness.
The engine leverages Precision RAG, a Retrieval Augmented Generation approach of prompting LLMs (Large language models) with a given question and context from which to provide their answer. Precision RAG has guardrails in place to ensure the relevance and correctness of the provided answer.
A search engine retrieves a list of relevant links or items most related to a given query which, in most cases, needs to be short and specific. An answer engine provides an actual answer to a given question, which can be long and complex, while noting the items this answer is based on.
Unlike most search engines employed in RAG setups, which employ a singular, or sometimes a small combination of models for the retrieval part of the process, xFind employs a broad setup of models which complement each other.
This enables Knowledge Copilot to:
- Successfully deal with queries much longer than classic search
- Successfully retrieve from more complex sources, such as past cases
- Be much more focused in retrieving the relevant items at the top of the list in order to feed the answering LLM
Retrieval Augmented Generation – an approach of prompting LLMs (large language models) with a given question and context from which to provide an answer.
Precision RAG provides trusted, real-time answers to complex questions, removing the need to deduce these answers from the various existing sources in which they reside.
Precision RAG is a method for turning complex queries and disparate data sources into precise answers – the ideal technology for handling support case data and complex customer questions.
Many don’t realize the complexity and challenges involved in developing AI-based question-answering solutions. They often underestimate the intricacies of handling unstructured data and ensuring accurate and cost-effective retrieval and response generation.
The main challenges include:
- Data source complexity: Enterprises often rely on unstructured data from past cases, tickets, emails, etc., which requires advanced parsing pipelines for consistency.
- Search inaccuracy: Pre-trained embeddings from models like OpenAI, Claude, Mistral, and LLama are not precise enough for domain-specific data, leading to inaccuracies in retrieval.
- Cost: Reducing costs at scale involves focusing queries only on necessary information, requiring a highly precise search engine.
- Search failure detection: It’s crucial to know when the system doesn’t have an answer, especially in high-stakes support scenarios, to manage customer expectations and provide accurate information.
The effectiveness of Retrieval Augmented Generation (RAG) in enterprise settings depends primarily on the quality of the search engine. A powerful search engine ensures precise and relevant data retrieval, which is critical for generating accurate answers, regardless of the generative LLM’s capabilities.
Knowledge Copilot leverages advanced technology to manage these challenges at scale, including:
- Data preparation pipelines: These pipelines can handle any data format and type, making it ready for both search engines and LLMs.
- Hybrid search engine setup: xFind integrates various language model types and methods, allowing for a plug-and-play setup with minimal time and no training data required.
- Guardrails: Real-time assessment ensures queries are relevant to the knowledge domain and that sources contain accurate information, minimizing hallucinations and errors.
The proprietary guardrails in xFind’s technology automatically evaluate whether a query is relevant to the knowledge domain and if the sources can provide an accurate answer. This helps minimize errors related to topic mismatches or missing data, ensuring more reliable and accurate responses.
Knowledge Copilot implements guardrails that are able to detect:
- If a query is in the general domain of the customer’s knowledge, to avoid answering irrelevant questions such as ‘how do I walk my dog’
- If the results retrieved include information that could be relevant to answering the question
- Focus the items retrieved to a very specific set, to help the LLM with finding the relevant information to use when building the response
Any textual knowledge source, including voice transcriptions. Out-of-the-box sources include:
- Salesforce
- Zendesk
- Mindtouch
- KnowledgeOwl
- StackOverflow
- Salesforce Knowledge
- Zendesk articles
- Atlassian (Confluence, Jira)
- Notion
- Paligo
- GetGuru
- Gitbook
- Magentrix
- HigherLogic
- Doc360
- Slack
- Sitecore
- MS Teams
New customers typically onboard and start seeing AI insights from core implementation within 45 days.
SupportLogic implementation is a short and straightforward process after initial implementation.
Implementation is a flat fee. Contact us for a price estimate.
SupportLogic employs a usage-based pricing model. We charge an implementation fee for each module and then the actions you take in the system incur charges based on usage and value. This ensures you only pay for the parts of the platform that you use.
Contact us for a full estimate of annual pricing based on your initial case volume and selected use-cases.
Explore Resources on xFind and Precision RAG
Elevate Your Support Experience
Reduce escalations and cut through backlog to increase customer retention and revenue